Many manufacturers are enticed by the prospect of owning their machine learning solutions, rather than relying on external vendors. At Fero Labs, we're frequently asked: “Can’t I just build this myself?”
In theory, yes. An in-house solution can provide a competitive advantage, creating a highly individualized solution that turns all your organization’s proprietary know-how into actionable improvements.
But the DIY reality often does not match the dream.
We built a Decision Tree to help you to understand if an in-house build is right for your organization. For more detail on building your own solution, keep reading.
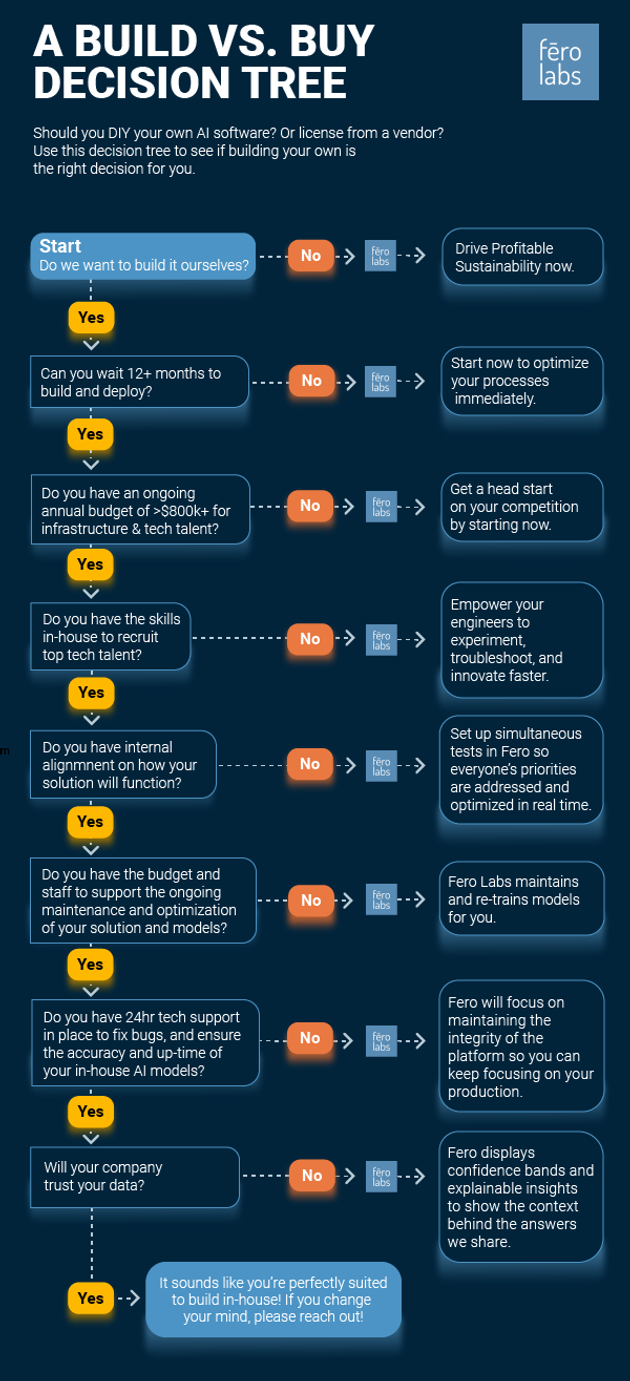
The Cost Conundrum
The costs of developing an in-house machine learning solution can be considerable. You’ll need to build hundreds of ML models, which means hiring product owners, data scientists, developers, and dev ops personnel. And once the solution is built, the models must be updated constantly based on production values in order to yield any value.
Value is critical. Consider machine learning a digital asset, just like the machines in a factory. To provide ROI, the value must be greater than the cost. Two out of three manufacturers report that they failed to realize ROI on their ML investments.
This suggests that they failed to take into account the total cost of ownership—which ranges far beyond initial coding investments, to knowledge transfer in case of personnel fluctuations, as well as software and hardware life cycle management such as dealing with tickets and change requests.
We advise manufacturers considering in-house solutions to think about the cost not only in terms of money but also time.
It takes a long time to develop and code an internal solution. Furthermore, the difficulty of communication between engineers and data scientists can stretch out already-lengthy product cycles. As the illustration below indicates, these can quickly add up.

The DIY Dream vs. The Vendor Alternative
We worked with a major international chemicals company that was trying to analyze a particular chemical process and predict when the catalyst would need to be cleaned or replaced based on operating condition trends. They’d developed their own models internally, which required a lot of code to maintain and had been built by a global team working for over a month to develop a standalone solution for the plant. Using Fero Labs software, a single engineer reproduced the ML models and user interface in 1.5 hours—no code required.
As this makes clear, saving time is a key benefit of choosing an external machine learning solution. A vendor like Fero Labs manages everything except hardware, so you don’t have to deal with huge personnel costs or internal processes—just install software.
Assuming the software has a way of encoding the specific process information relevant to the use case, this will also minimize pesky back-and-forth between engineers and data scientists, shortening project cycles significantly.
Find a Secure Vendor Solution that Adjusts to Your Unique Needs
There are always risks to relying on an external vendor. The software may not be individually fitted to your needs, like a custom-built solution. It’s important to take careful note of any prospective solution’s technology and feature set to make sure they can be applied to your use cases, and can adapt to your current and future needs.
Your data is your competitive advantage. When choosing a solution provider, be sure to select a vendor that trains their models only on your proprietary data, and doesn't share learning from one customer to the next.
Augment Your Experts with AI
If the reason you’re partial to building your own tool is that you already have data scientists and want to take advantage of their expertise, licensing an AI solution will augment them to work in better and different ways.
Licensing an AI solution will never replace your team of skilled experts. Think of AI as increasing headcount on every shift so your team can focus on more strategically imperative activities. Your software solution will help clean, capture and retain insights and outcomes faster making your team more collaborative and agile.
With software eliminating the time it takes to build models and clean data, your internal data scientists and process engineers can get to work on the tasks that are most closely aligned with what they were hired to do—solve the most complex problems to give you a competitive edge.